It is crucial to evaluate the data quality and source when making use of an AI stock trade predictor. This is because the accuracy of predictions directly depends on the integrity of data and its relevance. Here are the 10 best methods to evaluate data sources and quality.
1. Verify the accuracy and completeness of data
The reason: Accurate and complete data is foundational for building solid models.
How to check the accuracy by cross-checking data with numerous reliable sources (e.g. exchanges, financial databases). Verify that the data is complete by ensuring that there are no gaps or missing data points especially for data that is time-sensitive.
2. Consider the frequency and timeliness of data collection
Why: The stock market is highly fluid, and data that is not up to date can lead to outdated predictions.
How: Verify the data is being updated in real-time (or at a frequency which best suits your strategy) or on daily. In the case of high-frequency trading, or intraday trading, second-bysecond data might be required, while for long-term models, daily or weekly updates might suffice.
3. Examine the credibility and reliability of sources
Why: Reliable sources reduce the risk of using biased or inaccurate information, which may distort predictions.
Use only use data from reputable sources (e.g. Bloomberg Reuters NASDAQ) as often as is possible. Confirm the source is widely acknowledged and has quality control measures.
4. Make sure that you have consistency across sources.
The reason: inconsistent data could confuse models and reduce predictive accuracy.
Compare data from different sources and see if there is alignment. If one source consistently deviates look into possible issues such as variations in the methods of calculation or practices for data collection.
5. The scope of the data and the granularity of it
Why: The data must be granular and broad enough to capture all the specifics without introducing unnecessary noise.
How do you ensure that your data granularity corresponds to the timeframe of your predictions. In general, data from daily is sufficient to predict daily prices. However high-frequency models may require tick-level data. Make sure you include all relevant variables in the scope (e.g. the price of stocks, volume and economic indicators).
6. Look into the Historical Data Coverage
The use of data from the past is crucial for the development of robust training models and accurate backtesting.
Verify that the historical data includes several market cycles (bull, bear, and flat). This diversification enhances the model's ability to adapt to changing conditions.
7. Standards for Data Preprocessing Check
Why: Raw Data could be filled with noise or outliers that can affect model performance.
What methods should you be evaluating? used to clean and normalize data, such as how missing values and outliers are handled, along with any other transformations. Preprocessing is important to aid models in identifying relevant patterns, and not be affected by errors.
8. Assist in ensuring Regulatory Compliance
The reason: using non-compliant data could result in legal problems and fines.
How: Verify that the information is in line with relevant regulations (e.g. GDPR in Europe or SEC regulations in the United States). Check that the data doesn't contain private information or confidential information in the event that it is not anonymized.
9. Analyze data latency and accessibility
The reason: Even small delays in processing data for real-time trading could influence the timing of trades as well as profitability.
How to measure latency of data (delay from source to model) and ensure that it is compatible with the frequency of your trading. It is important to assess how quickly the data can be accessed, and whether this data can be seamlessly integrated with the AI prediction.
10. Consider Alternative Data to Gain Additional Insights
The reason: Other data sources such as news, sentiment, web traffic or social media can be used to improve traditional data.
How do you evaluate other sources of data that could enhance the insights of your model. Assure that these data sources are of a high-quality solid, reliable, and compatible with the model's input formats and also have a consistent design.
Following these guidelines will provide you with an excellent foundation to evaluate the data quality and the sources of any AI predictive model for trading stocks to help you avoid common pitfalls and ensure robust model performance. Check out the most popular ai stock market for more info including ai stock price, investment in share market, ai stock market, playing stocks, best ai stocks, playing stocks, stock market investing, ai stock trading, ai stock, best ai stocks and more.
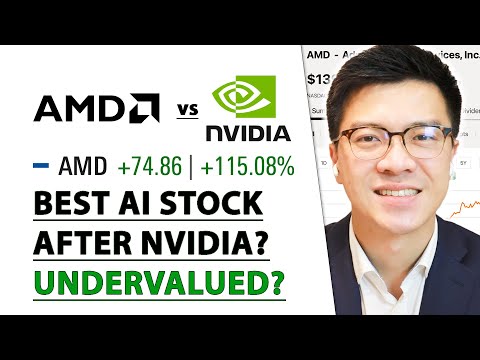
Top 10 Strategies To Help You Evaluate Tesla Stock By Using An Ai-Powered Stock Forecaster
To assess the performance of Tesla with an AI stock predictor, it is important to comprehend its business's dynamic as well as the market and any external factors. Here are 10 top tips to effectively evaluate Tesla's stock with an AI trading model:
1. Know Tesla's Business Model and Growth Strategy
The reason: Tesla is the leader in the electric vehicles (EV) and energy services markets.
How do you get familiar with Tesla's main business segments which include vehicle sales as well as energy generation and storage and software services. Understanding the company's growth strategy can help the AI model predict potential revenues streams as well as market share.
2. Market and Industry trends
The reason: Tesla's success has strongly influenced by changes in the world of automobiles and renewable energy.
How do you verify whether the AI model is taking into account relevant trends in the industry, such as the adoption rate of EVs and federal regulations. When comparing Tesla's performance against industry benchmarks, useful insights can be gained.
3. Evaluate the Impact of Earnings Reports
Why: Earnings releases can lead to large stock price fluctuations, especially in companies with high growth such as Tesla.
How to monitor Tesla's earnings calendar and examine how historical earnings surprises have affected stock performance. Integrate the advice provided by the firm into your model to help determine future expectations.
4. Technical Analysis Indicators
Why: Technical indicator helps detect short-term price trends and movements specific to Tesla's stocks.
How: Incorporate key technical indicators such as moving averages, Relative Strength Index (RSI) and Bollinger Bands into the AI model. These indicators can be used to identify potential entries and exit points in trading.
5. Macroeconomic and microeconomic Factors Analysis
Why: Economic conditions like interest rates, inflation, and consumer spending could affect Tesla's sales and profitability.
What should you do to ensure that the model includes macroeconomic indicators (e.g. the rate of growth in GDP, unemployment levels) and sector specific metrics (e.g. automobile sales trends). This context enhances the predictive abilities of the model.
6. Analyze Implement Sentiment
What is the reason? Investor sentiment is an important factor in determining the price of Tesla's shares, especially those who are involved in the volatile automotive or tech sectors.
How to: Use sentimental analysis of social media as well as analyst reports, financial news and other sources to determine public perception about Tesla. By incorporating this qualitative data the AI model can be given more context.
7. Monitor regulatory and policy changes
The reason: Tesla is a highly controlled field, and changes in government policies can impact the company's business.
Keep abreast of the latest policy developments pertaining to electric vehicles and renewable energy incentives, environmental regulations and more. Be sure to include these elements when estimating the effect of Tesla's activities.
8. Conduct Backtesting with Historical Data
Why is that the AI model can be evaluated by testing it back using previous price fluctuations and historical incidents.
How do you backtest the predictions of the model make use of historical data on Tesla stock. Comparing the outputs of the model to actual results will allow you to assess the accuracy and reliability.
9. Measure execution metrics in real-time
Why: To capitalize on Tesla's price movements it is essential to execute a plan.
What are the key metrics to monitor for execution, like gaps and fill rates. Analyze how well the AI model is able to predict the optimal times for entry and exit for Tesla trades. This will ensure that the execution matches predictions.
Review the size of your position and risk management Strategies
Tesla's volatility is a major reason why risk management is crucial to safeguard capital.
How to: Ensure the model includes strategies for risk management and position sizing in line with Tesla volatility as well as your portfolio risk. This can help reduce the risk of losses while also maximizing the return.
Following these tips can assist you in evaluating the AI predictive model for stock trading's capability to predict and analyze changes within Tesla stock. This will ensure that it remains accurate and current with the changing market conditions. Follow the top rated ai trading software url for site recommendations including ai stock investing, ai stocks to buy, stock market online, best stocks for ai, ai trading software, stock prediction website, ai stock trading app, chart stocks, ai copyright prediction, playing stocks and more.
